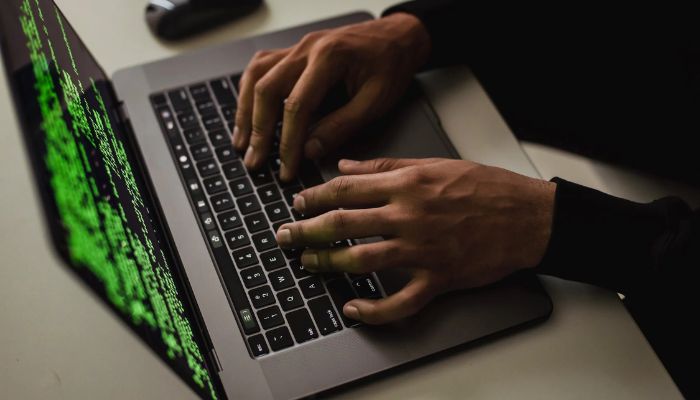
Synthetic identity fraud poses a formidable challenge in defrauding financial and commerce systems, with projected losses nearing $5 billion by 2024, according to Venture Beat. This type of fraud is notoriously difficult to detect and stop, eroding customer trust. However, artificial intelligence (AI) has emerged as a powerful ally in combatting synthetic identity fraud. Following are five key ways AI is contributing to the detection and prevention of this growing menace, as per AI writer Louis Columbus.
Key Contributions of AI
1. Embedding machine Learning at the core of fraud prevention
Achieving the delicate balance between catching fraudulent attempts and avoiding the alienation of legitimate customers is a crucial challenge for fraud systems and platform vendors. By leveraging machine learning (ML)-based algorithms that continuously learn from each fraud attempt, businesses can reduce false positives. ML and generative AI technologies are particularly well-suited for analysing real-time datasets of fraudulent activities, enabling constant improvement in authentication processes.
2. Incorporating ML into the code base
Preventing synthetic identity fraud across numerous retail locations necessitates ML-based platforms that continually learn and share insights derived from transaction data. By creating a fraud prevention ecosystem that expands its knowledge base, organisations can effectively combat this form of fraud. For instance, Splunk's approach to fraud risk scoring employs data pipelines for indexing, transformation, ML model training, and application, coupled with dashboarding and investigation tools. Proactive data analysis techniques significantly reduce the cost and duration of fraud incidents.
3. Reducing latency through Cloud Services:
Existing fraud prevention systems often suffer from longer latency compared to modern cloud services. Services like Amazon Fraud Detector, integrated with tools such as Amazon Cognito, offer tailored authentication workflows for identifying synthetic fraud activity. These fully managed cloud services have proven effective in identifying potential fraudulent activities, requiring no prior ML expertise from threat analysts and others.
4. Integration of user authentication workflows
Relying on numerous tools that lack integration hampers the ability to detect and respond to fraud alerts, overwhelming fraud analysts with multiple dashboards and reports. To enhance fraud detection, organisations require an integrated tech stack that can deliver ML-based efficacy at scale. The fusion of decades of transaction data with real-time telemetry data improves risk-scoring accuracy, enabling the identification of synthetic identity fraud before losses occur.
5. ML-based risk scores for enhanced onboarding
To strike a balance between fraud prevention and seamless onboarding, fraud analysts employ ML-based scoring methods that combine supervised and unsupervised learning. By reducing false positives through AI-based fraud scores, organisations minimise manual escalations, declines, and customer friction. This approach improves the overall customer experience while effectively preventing synthetic identity fraud.